Bedside Rounds
How do doctors actually think? And if we can answer that, can we train a computer to do a better job? In the post-WW2 period, a group of iconoclastic physicians set about to redefine the nature and structure of clinical reasoning and tried to build a diagnostic machine. Though they would ultimately fail, their failure set the stage for the birth of the electronic health records, formalized the review of systems, and set up a metacognitive conflict that remains unresolved to this day. This episode, entitled “The Database,” is the second part of this on the history of diagnosis with Gurpreet Dhaliwal.
First, listen to the podcast. After listening, ACP members can take the CME/MOC quiz for free.
CME/MOC:
Up to 0.75
AMA PRA Category 1 Credits ™ and MOC Points
Expires October 31, 2025
active
Cost:
Free to Members
Format:
Podcasts and Audio Content
Product:
Bedside Rounds
Bedside Rounds is a medical podcast by Adam Rodman, MD, about fascinating stories in clinical medicine rooted in history. ACP has teamed up with Adam to offer continuing medical education related to his podcasts, available exclusively to ACP members by completing the CME/MOC quiz.
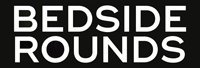
This is Adam Rodman, and you’re listening to Bedside Rounds, a monthly podcast on the weird, wonderful, and intensely human stories that have shaped modern medicine, brought to you in partnership with the American College of Physicians. This episode is called The Database, a continuation of my series on the history of diagnosis, and part two of this series with diagnostic guru Gurpreet Dhaliwal from UCSF.
So to quickly recap, by the second half of the nineteenth century, there was a workable, pragmatic model of both taking a history, and relating that information to the task of diagnosis: a synthetical method, where a complete “scientific” database of information is collected on every patient, which becomes the basis for further decisions, and an analytical method, where the patient’s complaint as well as the cognitive processes of the physician guide further questions and diagnostic maneuvers. The analytical method is generally preferred, both for logistical reasons – it takes a LOT less time – and for, well, reasoning reasons. We spent most of the episode talking about Jacob Mendez Da Costa’s Medical Diagnosis to explore these ideas. Da Costa clearly sees utility in both methods, but he presciently points: “In reasoning correctly on symptoms, the same laws apply as reasoning correctly on any other class of phenomena: the facts have to be sifted and weighed, not merely indiscriminately collected.”
This underscores a tension in history taking – and diagnostic thinking – that exists today:
Gurpreet Dhaliwal (22:45):
I think one of the things that reflected, it reminded me of a conversation I had, um, with one of my colleagues here, he has, um, he works in the subspecialty clinic, him and a, uh, fellow attending. They both have many decades of experience and they both have totally different approaches with the patients. Um, it's not quite the, the economies that we're talking about here, but one, a person is extremely structured. He actually really loves the orderly process of like HPI meds, uh, past medical history, uh, social history, health related behaviors in physical exam. And he follows that, um, which is not quite the synthetic method, but it is orderly. And it's definitely to make sure every piece of the database is in place, our modern database. And he has a colleague who basically chats up patients. He just sort of talks to them. Um, the, you know, the physical exam may happen.
Gurpreet Dhaliwal (23:29):
Then he might sit down and do some more, uh, meds. And then because of something that came out of the meds, he might say, you know, I'm gonna do this. So it has, if you mapped it out, it's just almost sarcastic and random. And he says, we both get to the same place with our patients. Now they're both in, they both now have many decades of experience, but it does inform the idea that there's, there's lots of ways to get to the right answer, whether the right answer is like the accurate diagnostic label or what the patient needs.
Gurpreet makes a couple good points here – but I think he’s very wise to point out his observations about what physicians actually DO, and not what we say we do. Because all of my arguments are based on textbooks, academic books, and journal articles. Textbooks should, after all, fundamentally be thought of as normative – they set expectations on what SHOULD happen, or rather, what the author thinks should happen – but often don’t reflect the realities of clinical practice. Think of it as the transition from the preclinical years to the medical wards – there’s often a huge culture shock as medical students encounter medicine is its messy reality. Unfortunately this discussion leaves perhaps the more pertinent question – how physicians in the late 19th century ACTUALLY talked with their patients to take a history – unanswered.
In any event, the scales would soon be tipped towards the ostensibly scientific “synthetical” method. The second half of the 19th century showed an increasing belief that the reams of data that bureaucratic states around the world were collecting could be used to gain deeper understandings about the world around us – including potentially previously hidden insights into how human beings work. This shouldn’t sound too unusual for you in the 21st century; we talk about large surveys and retrospective analyses all day and everyday. Log into Twitter right now and there will be dueling Twitter threads with inscrutable graphs about pretty much any topic you can think on the daily. This movement historically is generally known as positivism – the idea that things are true because of the positive – that is, they are defined by reason and logic from known facts. I actually spoke about the very early days of positivism in medicine in my episode on Florence Nightingale, about the influence of Quetelet. Positivist activists in the late 19th century took this empirical position even further, arguing that government policy and social policy (including, I should mention, eugenics) should fundamentally be driven in the same method – the mass collection of data, and then reasoning from that data.
Medicine – including clinical reasoning – was not immune from this movement. Da Costa’s analytical method in particular – dependent on the collection of data from a flawed human doctor from a flawed human patient – did not fit a growing expectation that medical thought be purely “scientific”. There is so much to say here – in fact, this is the predication of the entire movement of “scientific medicine” that would spread from Germany, across Europe and the Atlantic in the second half of the 19th century, in this country culminating in the Flexner report’s reforms to medical education. But these ideas were not just “inside baseball” – that physicians should be “scientific” was potent in the general sphere. I’m going to quote Bernard Shaw, who wrote an entire collection of essays called “Doctor’s Delusions,” that is deeply critical of modern, early 20th century medicine and its cognitive models.
“In my view,” he writes in the introduction, “surgeons and physicians should be primarily biologists. To tackle a damaged living organism with the outlook of a repairing plumber and joiner, or to treat an acid stomach by pouring an alkali into it, it to behave like a highly unintelligent artisan, and should entail instant and ignominious disqualification by the Privy Council.”
And Shaw had opinions about the tried-and-true methods of obtaining a history and making a diagnosis. Shaw writes an essay called “The Electronic Rayman”, a vaguely antisemitic essay about two quackish “diagnostic machines” that have been developed and marketed, naturally, and quoting here, by “Jewish doctors.” While being quite derisive of these ideas as currently formulated, Shaw nonetheless thinks them prescient. Quoting here:
“When I was young, big business offices employed hosts of bookkeepers, skilled in
writing and arithmetic, who, when the books came to be balanced, passed many distracted hours discovering and correcting their own errors. Clerks in those days had to make calculations of all
sorts, deducting rates from rents, income tax from dividends, ordinary discounts from ordinary accounts, or translating foreign into home currencies for money changing. Nowadays these
operations are performed by persons who need know neither how to write nor to add two and two correctly, by the use of calculating-cum-printing machines which are becoming as common as inkstands, though not quite so cheap. In the clinics and hospitals of the near future we may quite reasonably expect that the doctors will delegate all the preliminary work of diagnosis to machine operators as they now leave the taking of a temperature to a nurse, and with much more confidence in the accuracy of the report than they could place in the guesses of a member of the
Royal College of Physicians. Such machine work may be only a registration of symptoms; but I can conceive machines which would sort out combinations of symptoms and deliver a card stating the diagnosis and treatment according to rule.”
Of course, there are some doctors in whom he trusts clinical reasoning, but they are far and few between: “the proportion of practicing doctors possessing this instinct can hardly be more than ten per cent.”
Shaw, with a conviction that seems right out of the 21st century quality movement, understands how important making a diagnosis is: “With the rest the diagnosis follows from the symptoms; and the treatment is prescribed by the textbook. And the observation of the symptoms is extremely fallible, depending not only on the personal condition of the doctor (who has possibly been dragged to the case by his night bell after an exhausting day), but upon the replies of the patient to questions which are not always properly understood, and for lack of the necessary verbal skill could not be properly answered if they were understood. From such sources of error machinery is free.”
The essay was originally printed in the 19-teens; when collected in 1931, Shaw introduces a Czech word into this version introduced just a few years before: “To many readers this suggestion of Robot doctors operating diagnosing and prescribing machines may read like a reduction to absurdity of the routine of medical practice,” then giving a story of how his mother’s heart condition would have been far better treated by a “Robot doctor” with a capital R than the unfortunately very human one she received.
Gurpreet has been thinking about diagnosis for decades, and has seen interest in diagnostic machines ebb and flow. So I asked him what he thought about Shaw’s argument.
Gurpreet Dhaliwal (32:10):
I'm glad you asked that that's exactly what my response was. The idea that sort of, by a way of bookkeeping, um, we could, uh, move the data acquisition away from the doctor to something, another person or another machine. And then that calculations, instead of being in the doctor's mind can be done by a machine holds promise is a very familiar story. Now with computers and diagnosis, it sounds really reminiscent of it.
Adam Rodman (32:33):
Yeah. I know the fact that this is 104 years old, uh, just reading this quote and you're like, oh my goodness. None of, of these ideas are every, everything is old. Everything is,
Gurpreet Dhaliwal (32:41):
Everything is a remix. Yeah. Everything <laugh>, everything is remixed and, uh, revived.
Adam Rodman (32:46):
Gurpreet Dhaliwal (32:47):
Now I think it it's like the promise we have is the idea is that if, if we, you know, when we, uh, talk about, you know, remarkable advances that are made in machine learning, um, or computers these days in diagnosis, which I'm not Lu eye by any means, but I think there's a tremendous ways to go because the idea is if we can pour enough data into them, they can outsmart the human brain. Um, and that's the man versus machine, um, uh, prop that sort of gets brought out. I think most of us have moved on to the idea that, uh, person and machine is the way to go. Um, but there' is an outsized hope on what the machine can do.
Why would Shaw have so much hope on a far-future Robot? And why did he feel that only “10%” of practicing physicians were capable of actual diagnostic thought?
The depressing answer seems to be that this reflects the actual practice of medicine, and what was actually being taught. I will be the first to admit that this is an era that needs to be explored considerably more in the historical literature; most of the primary sources that I’ve found are from reformers complaining about their education years later. But Becker's Boys in White, an ethnographic study of students at the University of Kansas in the 1950s, paints a picture of clinical reasoning largely taught through “pearls,” aphorisms, and overarchingly “clinical experience.” I should also note that this is still a large part of teaching clinical reasoning – Kathryn Montgomery, who wrote one of my favorite books about clinical thinking, called “How Doctors Think” that Gurpreet and I talked about last episode, has an entire chapter about the use of aphorisms in medicine. The Flexnerian idealization of science in diagnostics was seemingly nowhere to be found.
Like so much in this series, fundamental steps to realizing all of this would be forged in war – WWII in particular, and coming from quite a surprising place: attempts to use new statistical methods to rapidly screen whether or not a draftee would be able to serve.
In particular, the military was deeply interested in screening out draftees with “neuropsychiatric disorders”, which according to their statistics costs the government 30,000 to 35,000 dollars per recruit. The Army alone reported 110,137 “neuropsychiatric casualties” in the US during WWI, at a rate of 26 per thousand soldiers. And to be clear, a good number of these were “shell shock,” what we might now call PTSD. A retrospective analysis of some of these discharges found that 83% of these soldiers had symptoms of their disorders prior to drafting; a government report found that “the majority of the psychiatric casualties we encountered could have been eliminated at the induction board if relatively simple social service data had been available.”
As the United States geared up to fight in the second World War II, the Army’s solution was to have a neuropsychiatrist evaluate draftees at the time of enlistment. Unfortunately, this was quickly becoming unfeasible. Preliminary studies showed that the most people a psychiatrist could evaluate was 10 men an hour, and no more than 50 men a day. At the rates the US was drafting young men to fight in the war, and with a pretty limited number of neuropsychiatrists, there was no way this was practicable.
A better method was needed. And to solve this problem, the Army turned to a team of physicians and statisticians – and while many different people worked on this, for the point of this story, the most important one was Keeve Brodman. I’m deeply indebted to Andrew Lea, a wonderful medical historian who wrote an essay in Isis about Brodman’s life during this period; the links are in the shownotes, and I will have an interview with him published in the coming months. Brodman was a young psychiatrist from New York City, part of the department of medicine at Cornell, when he was drafted into the Medical Army Corps. His specialty was the newly described “psychosomatic medicine.” Unfortunately, Brodman soon after developed multiple sclerosis – and as Lea points out, because of this, and his general home-bound status, he did much of his thinking via correspondence which survives to this day. He was discharged honorably from the military and returned to Cornell, where he continued to work on this project.
Brodman, who to be clear, at this point was just part of a larger team, and a junior member at that, co-developed their solution to the problem – the “Selectee Index.” It was made of three sections; the first a list of occupations, which the authors thought could predispose people to “neuropsychoses”. Which neuropsychoses? Their publication – along with the discussion afterwards – makes it clear that their main goal is to screen for homosexuality; their inspiration, quoting from the article: a previous study of homosexuals incarcerated at Rikers Island for “asocial” behavior, as well as other effeminate and homosexual subjects, demonstrated in a statistically reliable manner that certain occupations, e. g. interior decorator, dancer, window dresser, were chosen by these homosexuals with great frequency”. The second section was a basic personality inventory. The third – and for our purpose, the most interesting, was an inventory of symptoms. There were two types of questions – so-called STOP questions that would trigger an immediate evaluation by a neuropsychiatrist (for example, “have you ever had a fit?”) and more generic questions, such as “Are you easily discouraged”?
The research group validated the Selectee Index in “normal” soldiers and sailors, and then in groups about to be discharged for neuropsychiatric reasons. The results, from the Army’s standpoint, were remarkable – for example, the STOP questions were positive in 34% of soldiers discharged with a nervous breakdown, but less than 0.2 percent of the general population. And the score itself was highly predictive – if a soldier got a score of 20 or less, there was effectively no chance of a neuropsychiatric discharge. Ultimately, the Selectee Index – or Cornell Selectee Index as it was later known, was implemented at intake stations, though it wasn’t until very close to the end of the war. The index could be completed quite quickly by draftees; I read one account from an army psychiatrist that it could take between 5 and 15 minutes, depending on the savvy of the person completing it. He agreed with the authors of the original study that it was highly accurate for “weeding out” homosexuals, and he concluded:
“1. Use of the Cornell Selectee Index is an aid to the psychiatrist in forming a tentative, but usually accurate, opinion in a short space of time. 2. Obviously, it is not a substitute for a complete psychiatric examination, in itself. “
So I think there are two interesting points to make about this work. The first is that the Cornell Selectee Index is not at all interested in making diagnoses – it’s effectively a screening test to have a psychiatrist look in more detail. In fact, in the original piece in JAMA, the authors USE the language of screening, suggesting that it be used at the same time that soldiers would receive the Wassermann test for syphilis. And the second, which has not satisfyingly explored in the historical literature, is that fact that the military explicitly uses the relatively new language of sensitivity and specificity to talk about the test – a Lt. Cmdr William Hunt from the Navy quotes the above studies and compares the false positive rate of the Selectee Index to be 25%, compared to the 2% for a psychiatric interview.
After the war, Brodman continued his work, adapting the Cornell Selectee Index for civilian use. This new form was called the Cornell Index (I am sure you are noticing a theme in the names here); a large scale-validation based on “normal” patients and “disturbed” patients found a sensitivity between 72 and 75%, and a specificity between 83 and 87%. And to be clear here – he doesn’t explicitly use the words sensitivity and specificity; I just created a 2x2 table with his data and calculated it myself. Brodman is clearly excited about the performance of these tests – he suggests the test not only be used as an adjunct to taking a history in psychiatric patients, but also for widespread screening in medical wards and outpatient departments, in industry, and in returning veterans. He closes his paper with an enigmatic line about how all of this data might be used – it could be, he writes, “subjected to statistical analysis.”
There are two separate ideas here – one, does the Cornell Index work well as a screening test? The answer to that is “meh” – the sensitivity is low (in comparison, hepatitis C serologies have a sensitivity of around 98%), so there will be lots of false negatives. But the overall positive likelihood ratio is not bad, around 5.8. The other question is more philosophical – should we be screening in this fashion?
Gurpreet Dhaliwal (37:18):
So, right. Uh, thank you for that summary. I, you know, the, I feel when I hear it, I have a concern that maybe just pervades all screening efforts, right. We talk about screening for all sorts of important health issues, whether that's domestic violence or cancer screening, um, and all of the, um, them suffer from this sort of false, positive, false, negative, or sensitivity specificity. So if I had transported myself back in time, uh, I might have been, had the same concern here <laugh> that it, it may not perform well on either access or if it does good on one, it may not on the other, you know, we, uh, and we perform, you know, for instance, I work in an ER and we do, uh, screens for suicidality. Um, and, uh, it's a super important topic, especially important in the veteran's administration. One wonders if when someone is coming in with a, a cold or a gout flare, if you know, suicidality is the appropriate thing to be screening for. And, and same for many other things that get put into the triage approach.
Adam Rodman (38:15):
And I actually calculated out the sensitivity and specificity because I'm that type of nerd. Cause they have a, I made a two by two table that's surprised and it, it doesn't perform. I, the sensitivity was in the seventies. So, it doesn't perform that well. Right. I mean that, it performs really poorly as a screening test
Gurpreet Dhaliwal (38:29):
And for something that's as important as sort of, you know, fitness for the military, just like health of, of a patient, you have to decide is that tool worth doing, is that any better than the analytical approach?
Adam Rodman (38:40):
And after the war, they decided it wasn't, there's these huge, there's these huge debates where they, they, at this point they had a better language to actually talk about sensitivity and specificity and they ended up giving it up for those reasons.
Gurpreet Dhaliwal (38:50):
Yeah. I think they, the appeal upfront that we will sort of deconstruct something, score it and, uh, crank out a number that, uh, takes, you know, statistics are, uh, appealing, cuz they have this, um, uh, definitive to this, but they're never satisfying. Right. They never, like when you tell a patient there's an 82% chance of survival or something, uh, maybe it resolves some uncertainty, but there's a sense that it doesn't at all address the individual who's right in front of them. Patients certainly don't feel that way.
There’s no evidence that Brodman was swayed by this concern about screening – if anything, the possibility of using statistical methods to glean “deeper truths” inspired him to think even more ambitiously. Between 1947 and 1949, he led a project to develop an index that could be used for ALL symptoms, not just psychiatric ones – and more importantly, one that could theoretically be used for diagnosis. This index, called the Cornell Medical Index, was described in the initial papers as “a quick and reliable method of obtaining important facts about a patient’s medical history without expenditure of the physician’s time.”
Unlike the Cornell Index, where the goal was screening, to see if an individual needed further evaluation by a psychiatrist, the CMI was explicitly diagnostic in nature – the physician would have, even before meeting the patient, “information on which to base tentative diagnostic and prognostic appraisals of the patient’s total medical problem.”
It was intentionally designed to follow how a physician might interrogate the patient as a review of systems, with 195 questions divided by symptoms; answers were merely a yes or no. The different sections are organized by letters. The CMI took between 10 to 30 minutes to fill out completely, with 90% of patients completing it in less than 20 minutes.
The CMI was a huge hit – and moneymaker. At Cornell, every single patient who registered or came to an appointment filled one of these out until 1991. Cornell sold over a quarter million copies; it was used across the world, translated into numerous languages, and adapted for local usage. If you’ve ever filled out a “review of systems” form – or forced your patients to fill one out – these are all direct descendants of the CMI.
Here is where things start to get very interesting. The CMI is no screening test. It is meant to aid in diagnosis – though the user’s guide to the original CMI is careful to respect traditional physician autonomy in that the ultimate arbiter is the physician’s mind; the CMI is just a “rough sketch,” which has an interesting epistemological parallel to Da Costa’s painting imagery in the past episode.
Clearly many physicians over many decades found this helpful. So, I asked Gurpreet what he would have thought, if he were practicing circa 1950 and a patient with a cough handed this to him.
Gurpreet Dhaliwal (40:05):
I, I think not, uh, I, I wanna put away my bias from sort, you know, someone comes with a massive data load on me. I feel like, um, even if they say yes or no to certain things, almost everything requires context. So if they say they have cough, I have many questions that follow, you know, the character, the frequency what's associated with. If they say they don't have fever, but it wasn't me asking them. I don't know if they understood fever was subjective, feeling warm, uh, measuring on your thermometer. Um, so I think, you know, part of what we get from the questioning of course is the building of rapport. But part of the direct questioning we also get is validity of the data. Like I'm able to assure that when they say they didn't have a fever, what they meant is that actually I measured myself, my temperature every time and I never had a fever or temperature above 99.5. That's a very reassuring statement. The check box that says, I didn't have a fever is not. Um, so get back to analytical method of sort of, um, building truth with the patient rather than accepting it as a binary construct.
Adam Rodman (41:01):
Right. And so some of this is these things can't be dichotomized. Right. Um, and, and I will say that if you look at like Warner slack, there were future, uh, people who tried to build these review of systems on computers where it would have branching questions. So if they said no, or yes, it would ask, I would ask further. Do you think that would make it any easier? If there could be branching computers and put
But Brodman was thinking bigger than this. Even in the first publication on the CMI, Brodman is already interested in computerizing diagnosis: “Until a statistical method of scoring is formulated, the CMI, like medical data on a hospital history, is interpreted by clinical evaluation.” And in a trade publication, published the same year, Brodman voices these thoughts more explicitly, “Or will [the CMI] be used like a radio tube tester, and all by itself, in an attempt to make automatic diagnosis?”
Clearly Brodman had hoped that SOMEONE would use the data collected from the CMI to computerize diagnosis. By the early 1950s, he decided to do it himself. Again, for this section I am incredibly thankful to Andrew Lea and his scholarship in going through all of Brodman’s correspondence. Brodman noted that the data collected from the CMI was essentially a database – a large amount of diagnostic information on large amounts of patients, organized in a fashion that computers could understand, in all Yes/No questions.
Would this idea – branching Yes/No questions – provide more information? Gurpreet sees the promise.
Gurpreet Dhaliwal (41:21):
It questions? I think you, you can start to mimic the thought process of a clinician. What I said before about contextualizing a cough is not magic. Um, the only thing I can't guarantee is even if you sat with me in, you know, 20 clinic sessions and sort of mapped out how I dichotomize cough, um, it's conceivable that a 20, you know, on the 21st session, a patient will come in with some variable I have never thought about before, but I learned that they had traveled somewhere or they had an unusual congenital disease or there's a family history and it's not captured, but I actually know from the practice of medicine that, that has to influence the cough questioning. So, I think that it's conceivable for computers like those branching algorithms to capture what is known, but unless they get into, I guess what you call a machine learning algorithm where they learn from life itself, right? Um, they'll immediately become static and, and, uh, outlast or the, the real-world conditions will outstrip what they know.
Brodman was now thinking about mapping out physician cognition, and was way out of his league. He reached out to Robert Oppenheimer to see if he could recommend a mathematician “to derive a mathematical model of the operation of making diagnoses from the data, as an analogue to human thinking.” He hoped that such a model would shed truth about the human brain – “ [it] may also give information about the mechanisms of higher brain functions such as memory, concept formation, and judgment.” Oppenheimer dutifully replied, connecting Brodman with Julian Bigelow, one of the founders of cybernetics.
A brief aside on cybernetics. This is well beyond my expertise, but drastically oversimplifying – cybernetics was a multidisciplinary field that sought to define and describe self-regulating systems; the word comes from the Greek word for helmsman, imaging a sailor successfully navigating a ship through ever-changing environments. The word was invented by Norbert Wiener, another of the founders, who wrote an enormously popular book in the late 40s. Many of the disciplines we take for granted today – computer science, cognitive psychology, artificial intelligence, robotics, and systems science – all grew out of the cybernetics movement in the 40s and 50s.
In his first letter to Bigelow, Brodman lays out his theory of how diagnostic thinking works: “Some of the methods a person uses in reaching decisions for diagnoses are known. . . . For example, in determining which organ system is likely to be associated with a complaint on the questionnaire, the diagnostician unconsciously scans his memory. He estimates the frequency with which the complaint occurs with disorders in each organ system, and selects the one with which the complaint is most frequently associated.”
This sort of reasoning should sound quite familiar – this is a Bayesian spin on Da Costa’s synthetical reasoning. The physician collects a large database of information, and using probabilities, a list of diseases on a differential is calculated. As Brodman continued his collaborations with cyberneticists, he came down on his personal theory of diagnostics – pattern recognition. He wrote to van Woerkom, the cyberneticist who took over after Bigelow, “A physician certainly does not make computations when he makes a decision, nor does a person consciously use statistical techniques when he makes any decision. People recognize and manipulate patterns, gestalt. The model will do likewise.”
As Brodman was thinking about the medical mind, he was slowly accumulating the database in order to test his ideas. By 1950, he had over 5,000 completed CMIs from New York Hospital alone, which collated in a massive paper database. A pilot study soon followed – as Andrew Lea points out, done laboriously by hand – that looked at 25 common diagnoses that were already known from the chart, and looked at how symptoms on the CMI correlated to these. I don’t have access to this preliminary study – but it apparently was a success, or at least a proof of concept – that a mathematical model could be developed to fit symptom patterns to disease.
By the mid 1950s, as work on the project continued, a database of punch cards was developed; later it was transferred to magnetic tape. It grew ever larger – up to 23,000 entries. They started to use an IBM 704 to analyze the data – the massive room-sized computer that was the inspiration for HAL 9000 in 2001. In 1959, Brodman published his work to develop a diagnostic mind – what he would call Medical Data Screen, or MDS.
Published in JAMA, he opens with an excoriation on clinical reasoning: “the claim is frequently advanced that the process of drawing correct diagnostic inferences from a patient’s symptoms is in large part indefinable and intuitive;” alternatively, Brodman seeks to show that “the making of correct diagnostic interpretations of symptoms can be a process in all aspects logical and so completely defined that it can be carried out by a machine.”
Brodman was not only interested in tearing down the old model of clinical reasoning – he had a new definition: “First the system ascertains valid diagnostic criteria for the diseases it studies. By relating symptoms to the diseases in which they occur, the system determines for each disease the symptoms that have diagnostic significance. This information is stored in the "memory" of the machine. To make a differential diagnosis, the machine system compares the symptoms of a patient to the syndromes in its memory, computes the likelihood of the patient having any of the diseases about which it possesses information, and identifies the disease syndromes which the person's symptoms most closely resemble.”
To validate their new “Robot doctor” as Shaw might have called it, they evaluated the almost 6000 patients who had completed a CMI in the outpatient clinics at New York Hospital from 1948-1949, as well as ~ 3000 inpatients. These were compared against the patient’s age, sex, and most importantly, a list of diagnoses made by the clinical or hospital internists. The most common diagnoses, by the way, were “psychoneurosis,” inguinal hernia, prostate hypertrophy, and coronary artery disease.
Their machine diagnosed 44% of the 60 diseases, ranging from picking up 86% of kidney stones, to completely missing breast cysts. That might not seem great – but Brodman also studied the physician’s original diagnosis with the final diagnosis. The computer looks better here – in 15% of cases, the computer actually performed better than the physician, and the rates of “false diagnosis” were effectively the same between computer and human – about 6%.
The tone of Brodman’s piece is triumphant. “No a priori information based on the experience and knowledge of the medical profession” – the much-touted clinical judgment – was used he claimed; these disease syndromes were “discovered” independently by the machine. Mind you, this claim is clearly not true, since it was based on diagnoses that are often themselves derived from “clinical judgment,” with correlation coefficients that were manually calculated by very human authors making very human judgements.
Brodman is looking towards the future though. If the CMI works THIS well based only on patient-reported symptoms, imagine if we could add in even more data: “if, in addition, the machine had been given diagnostically definitive data from the physical and laboratory examinations, we would expect it to have been even more accurate in its diagnostic decisions.” Brodman is thinking big in his conclusion, but he understands his audience well – “a thinking person contemplating the same data and past experiences, can devise new methods of evaluating the data and can achieve previously unknown conclusions.” The machine, on the other hand “is merely a mechanical extension of the physician’s ability to remember, compare, compute, and decide.” Ultimately, though, Brodman’s MDS never took off. He started a company – the Medical Data Corporation – which sold a simplified version of the CMI intended to be used for large corporations to screen for diseases to keep health care costs down. But despite a cooperation with Roche, the company shut down in the early 1970s without ever making a sale.
Brodman did not succeed at his goals. I think that’s clear to everyone who practices medicine today – and was probably clear by the 1960s, as some of the hopes of this movement hit the brick wall of clinical – and computing – reality. I asked Gurpreet if he was surprised.
Gurpreet Dhaliwal (42:48):
I, I'm not surprised because I find diagnosis challenging. I think it's actually, it's incredibly challenging thing to do. And, um, I don't know if it's because the limits of knowledge and processing, right? We, we, um, sometimes say that's what computers can step in and fill the void on is the memory problems of the brain or the processing power of the brain or tendency towards biases. But I'm not sure if that's what makes the diagnostic process complicated. Um, if I go back to Montgomery's book, I think one of the things she emphasizes is why this is not a science is she's like you are taking this body of knowledge and adapting it for every individual patient. And the heterogeneity of patients is almost unbounded. Um, so it it's inconceivable that a computer program will be able to inconceivable. It's not the right word right now. It's not evident that a, a computer's gonna be able to do that part of the job, that part of the practice.
Adam Rodman (43:38):
And, and that's what struck me going back, right. Looking at what Keith Broadman is doing is effectively trying to use computers to do, uh, deco a synthetic method. It's the same sort of, of made a cognitive construct.
Gurpreet Dhaliwal (43:51):
Yeah. It, it, it's almost, uh, the, the synthetic method right. Takes, uh, you have to collect all of the data though, correct? Right. That in that synthetic method, if I come in with a cough and you go down the cough algorithm, you do then have to pivot and ask about abdominal pain, uh, and then switch gears and ask about back pain.
Adam Rodman (44:07):
Right. That's true. Right. There's still a human being doing it also.
Gurpreet Dhaliwal (44:10):
Yeah. So, but we don't do that right in our practice. So why, what is it that we know? Um, I think what happens is early in training, you, you may think that the, you know, the review systems is, is useful. And then you learn very quickly that the review systems is a dead end for 98% of patients. Um, and you learn that if I can just ask the few questions that round out, the story that's being built by the analytical method, that's the only review I need to do. It's filling in the blanks rather than having an endless series of blanks.
Adam Rodman (44:42):
Why do we teach the review of systems? Why do we stress? Like I know my medical they're shocks that I never, I never perform a review of systems. I do an I,
Gurpreet Dhaliwal (44:50):
I never either. I AC I actually don't know. I mean, you've educated me on the historical origins of how it came in. I think in some ways it's an artifact of these things that we, um, extol, which is like thoroughness. Um, you know, as we get, maybe later we'll talk about the history of clinical reasoning, but there was an error where we thought thoroughness was what accountant for expertise in diagnosis. And, um, thoroughness is still held as a virtue, right? How many times we say do a thorough H and P on your patient. Um, but when you study experts, they actually carry a very, they collect a very limited data, set a rather small and focused come out.
Adam Rodman (45:21):
So, they, they are able to figure out what is important, right? That's the, they can hone rapidly in what is important by the analytical method.
Gurpreet Dhaliwal (45:28):
I've also never modeled. I've never taught anyone to do a review systems, um, when they, when student does ask. And I think it's a great question. Well, why did you ask those follow up questions, you know, in the chest pain patient? Why did I ask, you know, about it being poetic and having taken a long plane ride and fevers? Uh, what I'm able to explain is when they, uh, say their chief concern is chest pain, I do open up a branching algorithm. I know we've talked about this sort of schema of different causes of chest pain. And if they're based on an anatomical structures, there are diseases at the end that are called illness scripts. And I do wanna make sure for the common and deadly diseases, I can, um, create a yes, no to their profile. So, if they haven't given me enough information, they say yes or no to the profile of pneumonia or yes or no to the profile of, um, aortic dissection, then I will ask questions. Um, and it may come across as a review of systems, but it's highly directed in the problem space of chest pain only
I think Gurpreet is onto something with Brodman’s legacy. Of course, we still don’t use computers to make diagnoses today for the most part, some 70 years after Brodman first started to think about this. The reasons why not are certainly interesting, and I’ll explore that in future episodes on diagnosis. He was probably the first to try though – decades later, Brodman was remembered by Howard Bleich as the first “proto-informaticist” – to try and build a diagnostic machine. His work on the CMI, of course, was incredibly influential – attested by the fact that it was used until 1991 at Cornell, and its descendants continue to be used to this day in the form of review of systems paperwork, though I’m not sure how happy that makes either doctors or patients.
But I want to make another suggestion here – that Brodman leaves us an indelible legacy, the first to try and redefine – or really, formally define – what it means to make a diagnosis. Let’s go all the way back to last episode, and the traditional clinical reasoning by way of Da Costa. Physicians can make diagnoses via the analytical method – where branching questioning and reasoning leads us to define a differential diagnosis. Or it can be made via the synthetical method, where a, dare I say it, database of standardized information is collected, and then diagnoses that fit this information are proffered. In designing his diagnostic machine, Brodman came down completely on the synthetical model. Data is collected – the CMI – and then analyzed by a computer algorithm that weights different symptoms by their frequency in diseases. It’s purely pattern recognition, and any failures in the MDS – which Brodman freely admitted – are purely the results of not enough data.
The same year that Brodman published his first results on the performance of the MDS – 1959 – a second paper was published in Science by Ledley and Lusted, probably the most important clinical reasoning paper of the middle of the 20th century, entitled the Reasoning Foundation of Medical Diagnosis. The paper is rather difficult to read; it relies on symbolic logic, conditional probability and Von Neumann’s theory of games – game theory was only a few years old at this point. But it lays out a theoretical model that runs on computerized punch cards of diagnosis. Patients would present with “symptom complexes” – combinations of historical data as well as diagnostic data. These would be compared with “disease complexes” – final diagnoses that have probabilities of certain findings associated with them. Just as is the MDS, Ledley and Lusted’s theoretical diagnostic machine would associate the patient’s “symptom complexes” with “disease complexes” and spit out the probability of a certain disease. Unlike the MDS, however, the probability of associations of symptoms (or findings) with certain diseases changes with every new patient, every new data point added to the system. “Each diagnosis, as it is made, itself becomes a statistic that changes the value of these probabilities,” they write. “Such changing probabilities reflect the spread of new epidemics, or new strains of antibiotic-resistant bacteria, or the discovery of new and better techniques of diagnosis and treatment, or new cures and preventive measures, or changes in social and economic standards, and so forth.” While they don’t credit him, Ledley and Lusted are clearly influenced by Yerushalmy’s work in integrating uncertainty into diagnosis.
Ledley and Lusted’s work promised a pattern-fitting diagnostic mind that didn’t require a single input from something like the CMI; rather, it could iteratively think as new clinical data was entered into its database. These ideas started to percolate just as cognitive psychology started to turn its attention to diagnosis. I’ll be the to admit that I don’t have a perfect understanding of the literature surrounding the psychology of modern clinical reasoning. Fortunately, I have Gurpreet.
Gurpreet Dhaliwal (46:31):
It's, you know, um, the illness script is, uh, sort of the third generation or fourth wave of clinical reasoning. Maybe if I can go back and just give a brief history of the 1970s on, um, cuz I know that we're melding these worlds, but you know, early on the 1970s was sort of the beginning of the study of clinical reasoning. You and I have discussed that it's clinical reasoning has been part of the profession forever, but I think it was the first inward analysis what's going on inside the clinician's mind. And the 1970s, um, really started McMaster university and Michigan state university with the idea that we could, uh, ask doctors to do think out loud protocols and by examining what, what comes outta their mouths, we can make some inference about what comes goes out in their mind. And that led to the idea of it being hypothetical deductive.
Gurpreet Dhaliwal (47:13):
That is to say that doctors generally hypotheses and they test and reject them. And while there was some truth to that, the process wasn't the magic because they just also discovered this concept called content specificity. Like I might be really good at forming hypotheses for rheumatologic diseases, but not so great at doing it for dermatologic diseases. And that's because there had to be something else. Um, then the 1980s came along and they thought maybe this is going to the key is memory like just raw memory, power and other virtuous things like thoroughness. Um, and that, you know, they had all these studies where they, um, saw they did memory tests like the, the great ones with chess masters, where they show a chess master and a chess novice aboard of 25 pieces. And the chess master could look at it after five seconds. And when they take it away, the chess master could recreate like 23 out of the 25 pieces.
Gurpreet Dhaliwal (48:00):
But the novice could only recreate maybe three or four of the pieces. And what they learned was that, you know, the, the chess master had knowledge organized in their brain, in these sort of chunks, there was some organization of knowledge, but what they found out was it was not raw memory power, cuz they also did these tests where they would even take attending physicians against medical students and just do tests of raw memory and guess who did better in a memory test and attending medical student medical by far? Yeah, yeah it does. They outstripped them, but they would find by the way, if the information was random, the medical student would do particularly better than an attending and recreating the details of the case. But if you gave the information in the history and physical and labs, the way it's organized, just like the chest grand master could remember the chunks, these specific moves or, or, um, um, plays on the chest board.
Gurpreet Dhaliwal (48:46):
The clinicians could understand like, oh, this sounded like endocarditis. Therefore, I can tell you the history physical exam. So, then the 1980s we came out of it were like, it's not raw memory power and it's certainly not thoroughness. They had figured out that people are experts get less information. They don't do the comprehensive review systems. And that led to, I think the 1990s and you know, we're, we're evolving from now, but the idea that there's these mental models that experts carry in their mind. Um, and there's two, you could describe. One is schema. The schema is when a problem happens. I have a structured approach to it every time. So, when how someone has chest pain, I know to take an anatomic approach and uh, that's for problems. But then when it comes to the diseases or the solutions to problems, the discovery or insight was made that these are encapsulated in our mind that these things called illness scripts.
Gurpreet Dhaliwal (49:32):
So these are imaginary files that we have in our mind. They're really sort of a network of neurons that say I have a profile in my mind of what pneumonia is like the history, the physical, the labs, the x-ray. And when we start school, we learn a very prototypical one. We have to it'd be too overwhelming if we didn't. Uh, but then what you learn is like the history slot has some variability in it. Some people have a cough, but not everyone. Some people have a fever, not everyone. Physical exam is variability labs and x-rays have variability, but you start to get this sort of, um, fuzzy image of like there's something called, uh, pneumonia and it's fit in this thing called an illness script. So short answer is the illness script is a memory structure that encapsulates our, uh, core knowledge and identification of a disease like pneumonia.
That was an absolutely amazing overview – and I’m not going to lie, I would listen to Gurpreet talk for three hours in a three-part episode on the intersection between cognitive psychology and diagnostic thinking. But this is already the longest episode in Bedside Rounds history, so we have to end somewhere. So we’ll end with Gurpreet and his takeaway of the cognitive development of the clinical database:
Gurpreet Dhaliwal (50:48):
I think that that idea, because I think now where this is coming up is not the doctor in their thoroughness, but whether the computer and big data's thoroughness is gonna replace the mind, I think that's the modern tension around this, that, um, uh, if we can just add more and more data, more and more coefficients, more and more waiting of, uh, um, and more and more experience, you know, feed the machine, the EMR it'll figure it out by itself. Um, and I, again, I, I, because of the complexity of what we do, that it's not a scientific task, no amount of modeling is gonna satisfy what this profession is asked to do. Um, because we have, we have to start from the individual patient and reason backwards and say, where can the can of medical knowledge help that person rather than, you know, rather than saying, like, I know a ton of stuff and I'm gonna make this person fit that mold.
Adam Rodman (51:39):
And, and that's, that's my takeaway also, right? I, my, my takeaway in, in looking into this is that the, the tension that we see now, that there are people in Silicon Valley wanting to replace doctors with machines. This has been around for over a hundred years. It's been around for 160 years. And the ideas that cognitive ideas behind them are old, they've been tested and they don't work because they missed the fundamental, uh, they missed what medicine is, right. They they're following not the Montgomery, they're following a positive view of what the practice.
Gurpreet Dhaliwal (52:08):
Yeah, very, very positive is. And this is not to say that medicine hasn't been transformed by technology for that same time. Right. What it does is technology just shifts the frontier of uncertainty. So, you know, it's sort of the same way people thought evidence-based medicine would resolve uncertainty in medicine. Um, and what it did was it, it, it informs judgment. Evidence based medicine informs our judgment, but it has very little role in replacing it. It improves it. You might move from random anecdotes to systematic studies of information, but you still apply it to the person who's in front of you. And the same will be true if a computer is thinking alongside of us, right.
That’s it for the show! Thank you so much for listening, and of course many thanks to Gurpreet Dhaliwal for his amazing insights into the nature of clinical reasoning. I’ve spent a good two years of my life doing a deep dive into this literature – and this episode represents only a small fraction! So there will be plenty more to come. My honest hope is that learning about the tensions in clinical thinking will help explain a little bit about how medicine ended up the way it has – and how it might continue to change in the future. So stay tuned!
CME is available for this episode if you’re a member of the American College of Physicians at www.acponline.org/BedsideRounds. All of the episodes are online at www.bedsiderounds.org, or on Apple Podcasts, Spotify, Google Podcasts, or the podcast retrieval method of your choice. The Facebook page is at /BedsideRounds. The show’s Twitter account is @BedsideRounds. If you want amazing Bedside Rounds swag designed by Sukriti Banthiya, the official merchandise stores is at www.teepublic.com/stores/BedsideRounds. I personally am @AdamRodmanMD on Twitter; I don’t always engage on Twitter, but when I do, you can bet it’s to argue about medical epistemology.
All of the sources are in the shownotes, and a transcript is available on the website.
And finally, while I am actually a doctor and I don’t just play one on the internet, this podcast is intended to be purely for entertainment and informational purposes, and should not be construed as medical advice. If you have any medical concerns, please see your primary care practitioner.
Contributors
Adam Rodman, MD, FACP
Gurpreet Dhaliwal, MD
Reviewers
Christopher Jackson, MD, FACP
Joshua Allen-Dicker, MD, MPH, ACP Member
Those named above, unless otherwise indicated, have no relevant financial relationships to disclose with ineligible companies whose primary business is producing, marketing, selling, re-selling, or distributing healthcare products used by or on patients. All relevant relationships have been mitigated.
Release Date: October 31, 2022
Expiration Date: October 31, 2025
CME Credit
This activity has been planned and implemented in accordance with the accreditation requirements and policies of the Accreditation Council for Continuing Medical Education (ACCME) through the joint providership of the American College of Physicians and Bedside Rounds. The American College of Physicians is accredited by the ACCME to provide continuing medical education for physicians.
The American College of Physicians designates this enduring material (podcast) for 0.75 AMA PRA Category 1 Credit™. Physicians should claim only the credit commensurate with the extent of their participation in the activity.
ABIM Maintenance of Certification (MOC) Points
Successful completion of this CME activity, which includes participation in the evaluation component, enables the participant to earn up to 0.75 medical knowledge MOC Point in the American Board of Internal Medicine’s (ABIM) Maintenance of Certification (MOC) program. Participants will earn MOC points equivalent to the amount of CME credits claimed for the activity. It is the CME activity provider’s responsibility to submit participant completion information to ACCME for the purpose of granting ABIM MOC credit.
How to Claim CME Credit and MOC Points
After listening to the podcast, complete a brief multiple-choice question quiz. To claim CME credit and MOC points you must achieve a minimum passing score of 66%. You may take the quiz multiple times to achieve a passing score.